Reliability
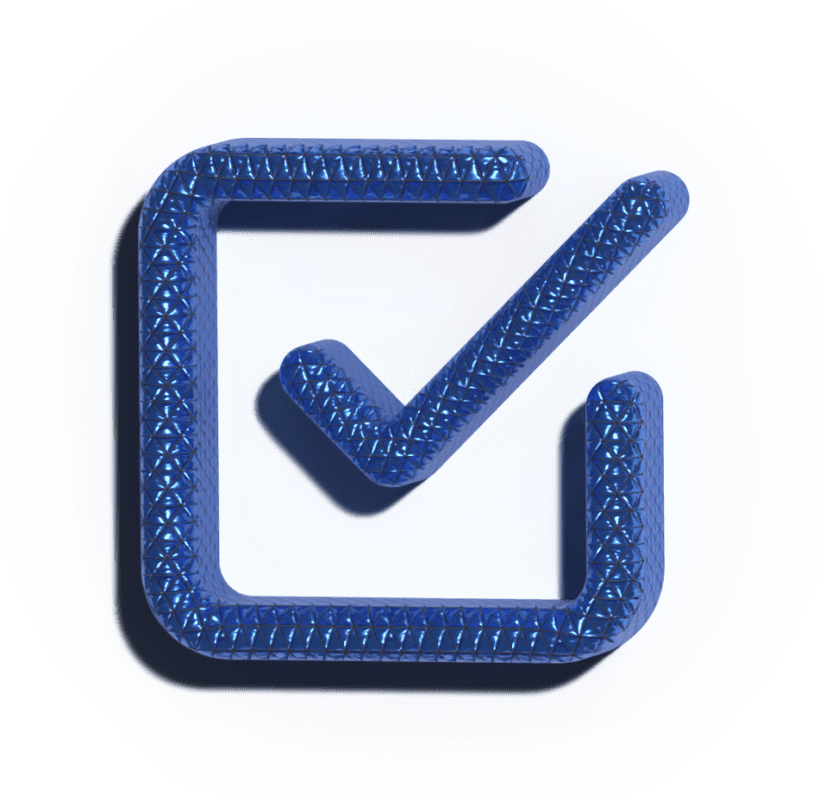
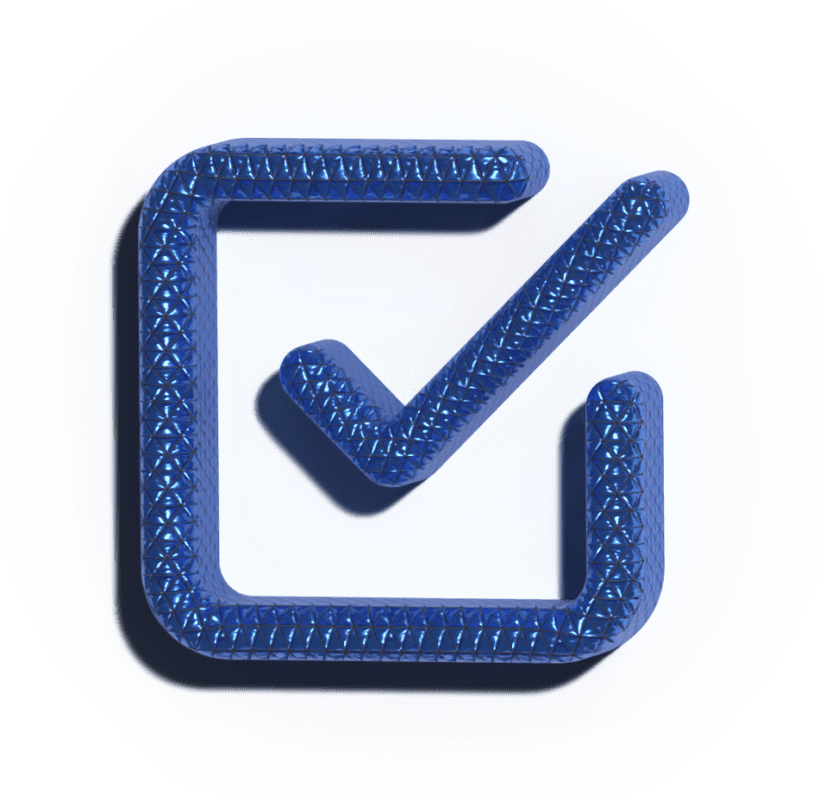
Ensuring Unmatched Reliability in AI for Business Operations
In the fast-paced world of business, the margin for error is minimal, and the cost of mistakes can be substantial. As companies increasingly integrate artificial intelligence (AI) into their operations, the reliability of these systems becomes paramount. A truly dependable AI system not only enhances efficiency and productivity but also fortifies the trust stakeholders place in automated processes. Recognizing this critical need, advancements in AI technology have led to the development of features designed to ensure unwavering reliability in every application.
RAG Technology: The Hallucination Preventer
One of the most innovative steps toward reliable AI is the implementation of RAG (Retrieval-Augmented Generation) technology. This cutting-edge approach addresses a common concern with AI: the tendency to “hallucinate” or generate plausible but entirely fabricated information. RAG technology mitigates this risk by grounding AI responses in verified data, thereby significantly enhancing the accuracy and trustworthiness of the information provided. This feature is especially crucial in scenarios where decision-making relies heavily on data integrity, such as in financial forecasting, medical diagnosis, and legal advisement.
Source Preview: Transparency in Information
Transparency is a cornerstone of reliability, and AI systems equipped with a Source Preview feature embody this principle. With every response or recommendation, the AI provides a preview of the data sources it referenced, allowing users to verify the information’s origin and accuracy. This level of transparency not only bolsters confidence in the AI’s capabilities but also empowers users to make informed decisions based on a clear understanding of the underlying data.
Auto Annotation: Highlighting What Matters
In the deluge of information that AI systems can process, identifying the most relevant data is crucial. The Auto Annotation feature addresses this challenge by automatically highlighting key information within sources, drawing attention to the most pertinent facts and figures. This capability ensures that users can quickly grasp the essential elements of the data, enhancing the efficiency and effectiveness of decision-making processes.
Chain-of-Thought: Unveiling the AI’s Reasoning
Perhaps one of the most transformative features of AI reliability is the Chain-of-Thought functionality. This feature allows users to follow the AI’s “thought process” step by step, understanding how it arrived at a particular conclusion or recommendation. By making the AI’s reasoning transparent, users can critically assess the logic behind the AI’s decisions, ensuring that they align with the company’s objectives and standards. This level of insight is invaluable, particularly in complex problem-solving scenarios where the rationale behind a decision is as important as the decision itself.
Conclusion – The integration of RAG Technology, Source Preview, Auto Annotation, and Chain-of-Thought functionalities into AI systems represents a significant leap forward in ensuring reliability in business operations. By addressing key concerns such as data accuracy, transparency, relevancy, and logical reasoning, these features collectively build a foundation of trust in AI technology. In an era where the stakes are high and the demand for efficiency is ever-increasing, investing in AI systems equipped with these capabilities is not just an option but a necessity for businesses aiming to thrive in the competitive landscape.